The term artificial intelligence in the automotive industry is almost synonymous with autonomous vehicles and intelligent ADAS features such as adaptive cruise control or lane-keeping assist. Since 2010, connected and autonomous vehicle development has attracted over US$200bn investment, with over 250 companies currently working on AVs and related technologies. However, with mounting pressure on OEMs to develop vehicles faster, with greater performance and safety, and all at a lower cost, the current use of AI only scratches the surface of how it can be used effectively to not only alleviate this pressure but empower engineers to design even better vehicles.
While there is an expectation that new cars will come with state-of-the-art autonomous, connected and/or electric technologies, engineering domain experts still need to create a fundamentally great car that boasts, for instance, theater-quality acoustics, a smooth driving experience in any environment and low emissions. Surprisingly, in spite of this mounting pressure, today’s process for designing and developing a vehicle from concept to production remains sequential, with built-in dependencies ripe with bottlenecks and delays that limit engineers’ creativity and slow down development, not to mention increase costs and risk market share losses from product delays and recalls. Moreover, although much of the vehicle design process includes innumerate processes that produce immense, highly valuable data, much of that data sadly goes unused or requires a team of data scientists to restructure and prepare the data set, once again keeping the engineer from doing her job – building a great car.
Engineering test data is competitively invaluable and represents a hidden treasure for the world’s automotive leaders. Visionary engineering leaders are beginning to realize that data from, for instance, wind tunnels or track tests can be used to train AI models to predict future aerodynamic performance without having to complete more tests in order to do so. By using test data to build self-learning models, vehicle engineering domain experts can instantly predict outcomes, explore more operating conditions and continuously improve vehicle design over time.
Machine learning for vehicle development represents a radical departure from existing methods in which combinations of simulations and physical testing are used to try and solve the mathematics of a highly complex, nonlinear system. Last year, AV companies Waymo and Cruise cumulatively drove 3.2 million miles in a bid to robustly train and test their autonomous tech. Engineering teams are hardly afforded this time anymore – competitors are moving too quickly – and yet the complexity of vehicle development has never been higher. When engineers struggle to understand the intractable physics of complex vehicle systems, such as airflow in aerodynamics, self-learning models can replace or complement solving the underlying physics with AI-based statistical predictions. Automotive teams at BMW, Honda and others are using Monolith software to take existing engineering data from simulations or physical tests to build self-learning models that instantly predict further test results or unearth new insights buried within the data.
These teams are substantially reducing the number of physical tests and simulations required during vehicle development, in turn reducing the time and cost of development by as much as 80%. Race team Jota used Monolith to reduce the number of simulations and tests by 50% and associated costs by 66%; put another way, they now get double the test time at two-thirds of the cost.
More and more companies are emerging in this space, allowing automotive companies to streamline development. For example, San Diego-based car company Aptera Motors used generative design techniques to optimize the chassis structure of its solar-electric vehicle for weight and crash performance.
Despite the promise of shorter and more cost-effective design cycles, introducing new tools into an age-old product development process requires vision, courage and experimentation. That experimentation is made much easier when engineers understand that AI isn’t a complete replacement for simulations and physical tests – rather, it’s the vital last piece of the puzzle to enable engineers to reach design convergence much more quickly and efficiently. Veteran engineers will have experienced a similar transition when simulations were first introduced to the workflow. This eventually meant engineers didn’t have to go back to first principles with every design change. Introducing AI is a similar step change – suddenly engineers don’t have to solve the physics underpinning a mathematical system but can now instantly unleash the insights already hidden in the data.
It’s also important to find the right use case for AI in vehicle development. Using statistical predictions over physics-based modeling makes sense for complex systems in which the accuracy of simulations is limited by the discretization of the physical domain. Some examples of complex vehicle systems that can benefit most from AI include aerodynamics, thermodynamics, track tests, wheel and tire degradation, vehicle dynamics, durability, and crash and powertrain testing. The accuracy of solving non-linear equations such as Navier-Stokes is determined by parameters like the number of simulation iterations, the granularity of time steps and the mesh resolution. Results are therefore a representation of the real world and must be calibrated and validated via extensive physical testing. With the right data input, artificial intelligence can close the gap between the real-world physics and the representations made in simulations, offering engineers more accurate and instant results.
Aerodynamics is a clear example of this approach. Every vehicle program requires aerodynamics engineers to spend thousands of euros in the wind tunnel, having already completed hundreds of simulations, a process that will be repeated many times during the vehicle’s development. By integrating Monolith self-learning models into the workflow and training them on aerodynamics engineers’ data, engineers get instant insights to point them in the right direction toward only the most strategic tests. In working with the world’s top engineering teams to integrate Monolith into their engineering product development workflows, it is as game-changing as equipping an explorer with a satnav instead of navigating by the stars.
The opportunity to reduce simulations and physical tests and in turn accelerate development makes a clear case for using artificial intelligence upstream in vehicle design and development. However, realizing the true potential of left-shifting design convergence by using AI can only be achieved if it’s matched by the apparently smallest change to the current engineering workflow. Monolith’s AI platform has been designed with a laser focus on user experience so it can seamlessly drop into existing processes and start giving engineers valuable insights and valuable time back from the get-go.
The race for electrification will no doubt force automotive companies and Tier 1 suppliers to find innovative new solutions in order to remain competitive. Implementing artificial intelligence throughout the automotive development process is a great place to start.
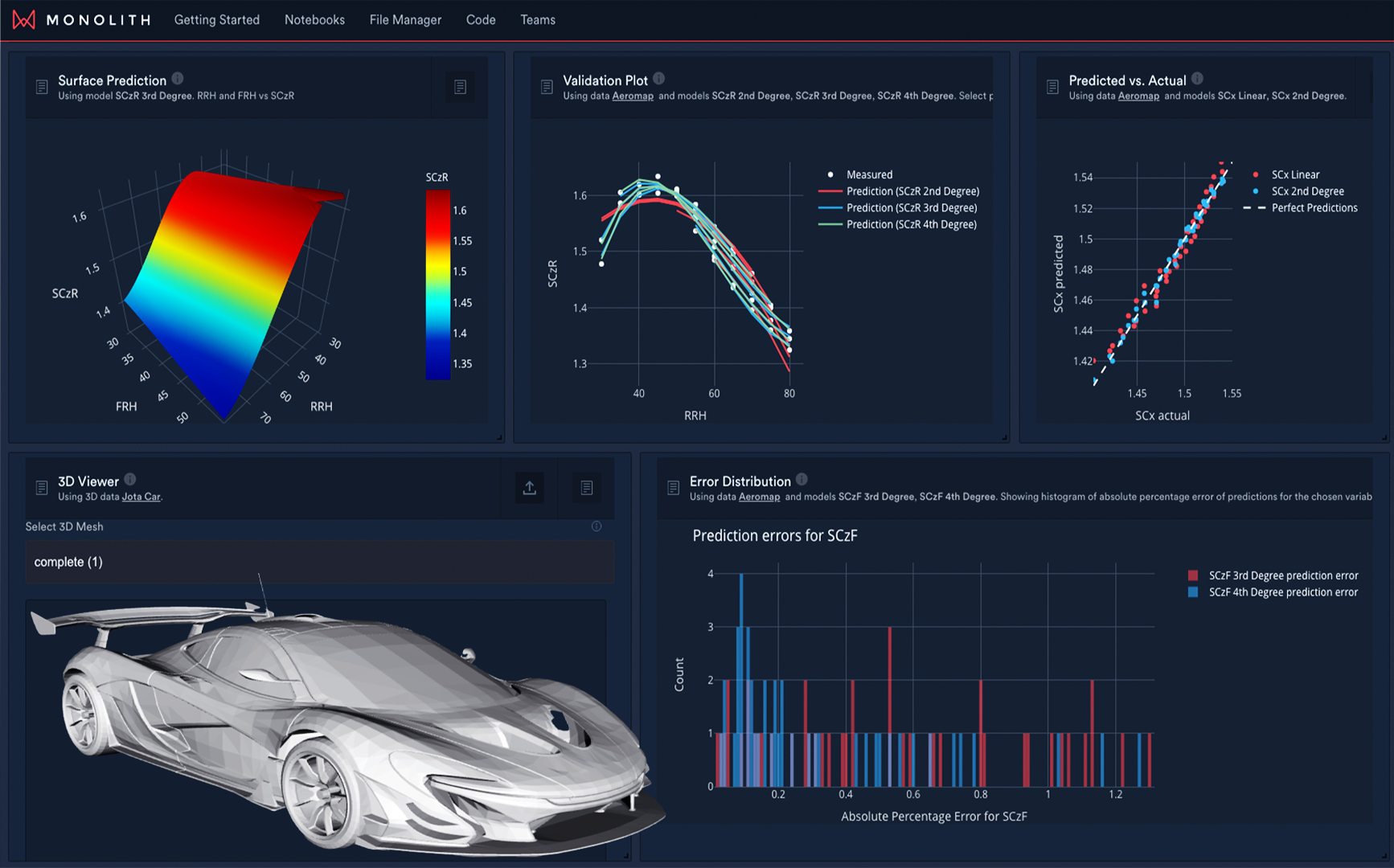